Why Teach with Data?
To teach with data usually requires curricular revision and a willingness to give up some control of the classroom. So, what's the upside? While there are no doubt many more reasons than these, below are six reasons most commonly cited by education experts:
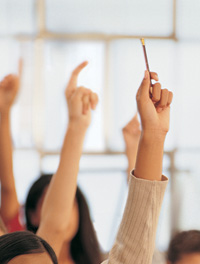
- As an active learning strategy, teaching with data increases retention
- Working with data introduces students to problem-solving techniques for the messiness of real-world problems
- Teaching with data supports critical quantitative reasoning capacities
- Teaching with data can teach modeling skills
- Working with real data raises questions of ethical research
- Hands on research shifts attitudes toward science and quantitative analysis
1. Active learning strategies increase retention
Interactive engagement -- the involvement of students in active learning strategies -- has been shown (in general) to improve student mastery of content knowledge and retention of material over time. And many teaching with data activities put students in an active role.
David Lopatto (2004, 2006, 2007, 2010) has extensively studied the benefits of research activities as a particular form of active learning. (For the sciences, see Hunter et al. 2007, Laursen et al. 2010, Meltzer and Thornton 2012, and Sadler et al. 2010.) While Lopatto's work originated in the context of the sciences, it also includes study of student experiences in the social sciences and humanities (see also Craney et al. 2011). Similarly, while some of the analysis is of summer research experiences, very similar results are found when studying "research like" experiences in the classroom (Denofrio et al. 2007 and Lopatto 2010). That description certainly fits teaching with data.
What this literature finds is that engagement in active learning:
- Increases student learning retention: They will remember what you teach longer!
- Nurtures persistence through the major (at least in the sciences)
- Improves aptitude in disciplinary methods/skills
- Fosters a deeper understanding of the process of doing research.
2. Data are messy and complex...just like the real world
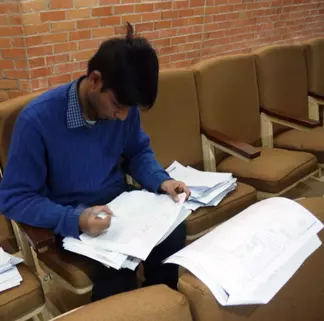
The messiness of data also give students practice in transference. Education expert Grant Wiggins shares an experience he had coaching soccer. After meticulously drilling his team, he noted that they were still unable to implement the 3-on-2 attack they had practiced. Even as he called out to them to help them recognize the situation, they were stymied. His co-captain replied in frustration, "I can't SEE it now; they won't line up like the way we did the drill!"
If we practice only in the most sterile conditions, our students will not acquire the ability to see through the noise to the underlying structures and processes we want them to learn. Teaching with data confronts students with (manageably) muddled contexts in which to grow these transference skills.
3. Teaching with data promotes critical quantitative reasoning skills
Quantitative reasoning-- the habit of mind to apply data and quantitative tools to a wide range of problems in personal, professional, and public contexts -- is among key learning outcomes for 21st century students. For example, in its LEAP initiative the Association of American Colleges and Universities names QL among 6 "Intellectual and Practical Skills" necessary to "prepare for twenty-first-century challenges."
In the United States we have good reason to doubt that we are preparing our students for this world. For example, the OECD's Programme for International Student Assessment (PISA) finds US high schoolers in the middle of the pack -- ranked 31st out of 65 countries in QR's cousin, mathematical literacy. Teaching with data provides students an excellent opportunity to develop the capacity to access, manipulate, analyze, and present quantitative evidence. For more on teaching quantitative reasoning, consult this detailed module.
4. When the data used are simulated, students learn valuable modeling principles
Like other forms of teaching with data, modeling shares in many of the broad advantages of teaching with data while contributing benefits specific to its particular form. One of the greatest benefits of teaching with models is that it explicitly requires students to consider the assumptions underlying their analysis (Starfield and Salter 2010 p. 117).
Of course, assumptions are critical to all types of data work. The unbiased nature of statistical estimates relies on a set of assumptions. The meaning of survey averages is dependent on assumptions about sample construction. Even basic information on counts of one thing or another has meaning only under particular assumptions concerning the definition of the measured concept. What is different about modeling is that it forces students to articulate their assumptions and then encourages them to perform sensitivity analyses by changing those assumptions.
This experience confronts students with a new kind of uncertainty--the uncertainty of model construction. In a world in which computers quickly estimate regressions based on millions of observations, this model uncertainty is likely of greater importance than uncertainty due to chance in sampling. A good lesson for our students to learn!
5. Analyzing data provides opportunity for reflection on ethical research practices
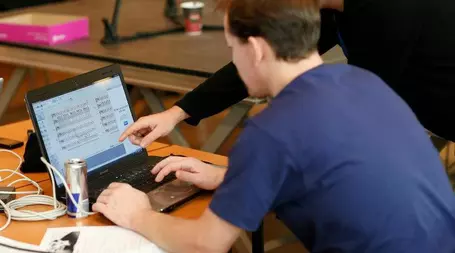
While working with data inherently involves ethical choices, these choices are not necessarily apparent to students without explicit guidance. Lopatto (2006), for example, reports that, while summer research experiences (in social sciences and humanities as well as the sciences) produce sizable gains in many dimensions, "[a] more sobering finding was that the least gain was in 'learning ethical conduct'." Lopatto notes this result has also been found in previous examinations. This finding suggests that teachers must prompt students to recognize the ethical aspects of their research choices if this benefit is to be realized. Perhaps this explains why Lopatto (2010) reports greater self-reported gains in ethical conduct in classroom-based research-like experiences than in summer research.
6. Hands-on research experience improves attitudes toward science and quantitative analysis
The work by David Lopatto and others cited in the first section above also suggests that students who have research-like experiences (such as working directly with data) alter students perceptions of research. Not only do they have a better idea of what research actually is, they also have a more positive attitude toward that process and their own abilities. Thus, a guided classroom experience of teaching with data can have long-term spillovers as students mature and have opportunities to do independent work.
References
Best, Joel. 2004. More Damned Lies and Statistics: How Numbers Confuse Public Issues. Berkeley, CA: University of California Press.Craney, Chris; Tara McKay; April Mazzeo; Janet Morris; Cheryl Prigodich; and Robert de Groot. 2011. "Cross-Discipline Perception of the Undergraduate Research Experience," Journal of Higher Education, 82(1): 92-113.
Denefrio, Lauren A; Brandy Russell; David Lopatto; and Yi Lu. 2007. "Linking Student Interests to Science Curricula," Science, 318: 1872-1873.
Hunter, Anne-Barrie; Sandra L. Laursen; and Elaine Seymour. 2007. "Becoming a Scientist: The Role of Undergraduate Research in Students' Cognitive, Personal, and Professional Development," Science Education, 91(1): 36-74.
Laursen, Sandra; Anne-Barrie Hunter; Elaine Seymour; and Heather Thiry. 2010. Undergraduate Research in the Sciences: Engaging Students in Real Science. San Francisco, CA: Jossey-Bass.
Lopatto, David. 2004. "Survey of Undergraduate Research Experiences (SURE): First Findings," Cell Biology Education, 3(4): 270-277.
Lopatto, David. 2006. "Undergraduate Research as a Catalyst for Liberal Learning," Peer Review, 8(1): 22–25.
Lopatto, David. 2007. "Undergraduate Research Experiences Support Science Career Decisions and Active Learning," CBE Life Sciences Education, 6(4): 297-306.
Lopatto, David. 2010. "Undergraduate Research as a a High-Impact Student Experience," Peer Review, 12(2): 27-30.
Meltzer, David E. and Ronald K. Thornton. 2012. "Resource Letter ALIP–1: Active-Learning Instruction in Physics," American Journal of Physics, 80(6): 478-496.
Sadler, Troy D.; Stephen Burgin; Lyle McKinney; and Luis Ponjuan. 2010. "Learning Science Through Research Apprenticeships: A Critical Review of the Literature," Journal of Research in Science Teaching, 47(3): 235-256.
Starfield, Anthony M. and Richard M. Salter. 2010. "Thoughts on a General Undergraduate Modelling Course and Software to Support It," Transactions of the royal Society of South Africa, 65(2): 116-121.