The Evolution of Pearson’s Correlation Coefficient/Exploring Relationships between Two Quantitative Variables
Gary D. Kader
Department of Mathematical Sciences
Appalachian State University
Boone, NC 28608
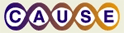
This material was originally developed through
CAUSE as part of its collaboration with the
SERC Pedagogic Service.
This activity has been undergone anonymous peer review.
This activity was anonymously reviewed by educators with appropriate statistics background according
to the CAUSE review criteria for its pedagogic collection.
Initial Publication Date: January 10, 2008
Summary
Using interactive lecture, this activity explores a collection of nine scatterplots to develop the notion of association between two quantitative variables. The activity is designed to help students better understand how statistical measures are "invented," and why certain measures are preferred. Specifically, this activity proposes a non-standard "intuitive" measure of association and, by examining properties of this measure, develops the more standard measure, Pearson's Correlation Coefficient.
Topics
Data Presentation, Statistical Inference and Techniques, Statistics, Mathematics
Grade Level
High School (9-12), College Introductory, College Lower (13-14)
Share your modifications and improvements to this activity through the Community Contribution Tool »Learning Goals
Students will come to understand the notion of association between two quantitative variables and Pearson's Correlation Coefficient as a measure of the direction and strength of the linear relationship between two quantitative variables.
Context for Use
This activity is usually done as an introduction to the study of relationships between two quantitative variables. The lesson takes approximately two class periods (100 minutes) and works best as an interactive lecture. The activity is designed for introductory statistics at the high school or college level.
Teaching Materials
The activity explores a collection of scatterplots. With the exception of the first two scatterplots, the data were constructed to control for characteristics that students might attend to when judging the direction, form and strength of the relationship between the two variables. A detailed description of the activity, including the scatterplots and discussions, will be available in NCTM's Mathematics Teacher Focus Issue on Data Analysis and Probability, November 2008.
Teaching Notes and Tips
Introduction to Bivariate Data and Association
The first part of this activity is an interactive lecture using whole group discussion of a scatterplot to understand association. Below is an example of this discussion including scatterplots, questions and prompts.
Introduction
Today we will examine a problem from anthropometrics, the statistical study of the human body and relationships between difference human characteristics. Specifically, we will explore the following statistical question:
-
Is there a relationship between arm span and height?
Prompts for discussion"
- What do you think?
- Do short people generally have short arms?
- Can short people have long arms?
- Do tall people generally have long arms?
- Can tall people have short arms?
You might collect data on height and arm span for the students in your class or
you can download data (Acrobat (PDF) 24kB Oct18 07) for Example 1.
You can create a scatterplot for the data collected in class or
you can download the
scatterplot (Acrobat (PDF) 53kB Oct30 07) for Example 1.
Based on the scatterplot how would you describe the relationship between height and arm span? Some specific questions to address include:
-
How would you characterize the arm span for the shorter people in this study?
- How would you characterize the arm span for the taller people in this study?
-
Based on the scatterplot, is it always true that if one person is taller that another person, that he/she will have longer arms? Explain.
-
Is the plot of the data perfectly linear? Is it generally linear?
-
How strong is the relationship between height and armspan?
The Quadrant Count Ratio: A First Measure for Strength of Association
The quadrant count ratio (QCR) provides a measure of the strength of association between two quantitative variable. To determine the QCR, the scatterplot of the data is divided into four "quadrants" based on the mean values of the two variables. This idea is illustrated in the
scatterplot (Acrobat (PDF) 89kB Oct31 07) for the height-armspan data.
The QCR is defined to be:
[(The Number of Points in Quadrants I and III) - (The Number of Points in the Quadrants II and IV)]/[The Total Number of Points]
From the definition, the value of the QCR is guaranteed to be between -1 and 1, inclusive, and the QCR does not depend on the units of measurement for the two variables.
Additional properties of the QCR are best explored through scatterplots. In the following examples, there is no context. The illustrations are designed simply to demonstrate various properties of the QCR. Each scatterplot has been divided into the four quadrants based on the means. Each scatterplot is followed by questions that should be addressed by students. A discussion related to these questions is provided following each scatterplot. As properties of the QCR evolve, they will be noted.
Example 2 (Acrobat (PDF) 53kB Oct23 07)
The trend is generally negative. Most of the points are in Quadrants II and IV, which supports the statement of generally negative association. There appears to be a fairly strong linear relationship between the two variables. QCR = [(1+2)-(12+10)]/25 = -.76. The QCR is negative and suggests a fairly strong negative association between Y and X.
Property 1:
If the points are predominately in Quadrants I and III, then the QCR will be positive.
If the points are predominately in Quadrants II and IV, then the QCR will be negative.
OR
If the association is positive, then the QCR will be positive.
If the association is negative, then the QCR will be negative.
The stronger the association, the closer to ???1 the QCR will be.
Example 3 (Acrobat (PDF) 54kB Oct23 07)
There is no general trend. Each of the four quadrants has about the same number of points. There does not appear to be any relationship between the two variables and the association is generally week. The QCR = [(8+6)—((4+7)]/25 = .12. The QCR is positive, but close to 0, suggesting a fairly weak positive association.
Property 2:
When the association between the two variables is weak, the QCR is close to 0.
Example 4 (Acrobat (PDF) 52kB Oct23 07)
The trend in the scatterplot is perfectly positive and perfectly linear. All the points are in Quadrants I and III and the QCR = 1.
Property 3:
When the relationship is perfectly linear, the QCR will be ???1.
When there is a strong positive (negative) association, the QCR is close to +1 (-1). When there is little association, the QCR is close to 0. When all the points are on a line, the QCR will be ???1. Consequently, a QCR close to ???1 suggests a strong association, while a QCR close to 0 suggests a weak association.
Thus, the QCR appears to behave the way we want in terms of characterizing the direction, form, and strength of the relationship between two variables. Unfortunately, since the QCR is a rather crude measure, it does not always provide the information we seek from a correlation coefficient as the following examples illustrate.
Example 5 (Acrobat (PDF) 44kB Oct23 07)
The trend in the scatterplot is perfectly positive but not perfectly linear. All the points are in Quadrants I and III and the QCR = 1.
Property 4:
A QCR = ???1 does not mean the relationship between the two variables is perfectly linear.
Note that in this example, the data satisfy Y = X^2.
Example 6 (Acrobat (PDF) 53kB Oct23 07)
The trend in the scatterplot is generally positive and linear but it is not perfectly linear. However, all the points are in Quadrants I and III and the QCR = 1.
Property 5:
When all the points are in Quadrants I and III then the QCR will be 1.
When all the points are in Quadrants II and IV then the QCR will be -1.
Consequently, the QCR can be ???1 even when the relationship between Y and X is not exact.
In example 7 two scatterplots are compared to illustrate the primary weakness with the QCR. Note that the two scatterplots have the same scale.
Example 7 (Acrobat (PDF) 59kB Oct23 07)
Based on the data in Example 7a, there is no association and the QCR is 0.
However, in Example 7b, the data suggest a fairly strong positive linear relationship between Y and X. However, the QCR for these data is also 0.
These two examples point out the primary weakness in the QCR. All points have the same weight when determining the QCR. Each point has the same weight. Consequently, in Example 7b, even though the two points in QI and QIII are further away from the lines dividing the data into the four quadrants, they carry the same weight as the corresponding two points in Example 7a, which are closer to the lines.
Pearson's Correlation Coefficient
Pearson's r, takes into account how far each point is from the dividing lines and addresses this weakness in the QCR. Properties of r are explored through scatterplots. The seven illustrations used previously to devolop properties of the QCR will be re-examined. We will begin with Example 7 and then revisit Examples 1 through 6. Both the value for the QCR and Pearson's r will be reported for each scatterplot followed by a discussion on properties of r suggested by the scatterplot.
Example 7 Revisited (Acrobat (PDF) 47kB Oct23 07)
This example contrasts Pearson's r with the QCR and illustrates that points in Quadrants I and III have more weight in the determination of r.
Example 1 Revisited (Acrobat (PDF) 44kB Oct23 07)
Example 2 Revisited (Acrobat (PDF) 41kB Oct23 07)
In Example 1, there is a fairly strong positive linear trend, and the r is fairly close to 1.
In Example 2, there is a fairly strong negative linear trend, and the r is fairly close to -1.
Property 1: -1 ≤ r ≤ 1
When the general trend in negative, Pearson's r will be negative.
When the general trend in positive, Pearson's r will be positive.
Example 3 Revisited (Acrobat (PDF) 41kB Oct23 07)
There is little trend and r is fairly close to 0. .
Property 2:
When the association between the two variables is weak, then Pearson's r will be close to 0.
Example 4 Revisited (Acrobat (PDF) 40kB Oct23 07)
The relationship is perfectly linear and Pearson's r is 1.
Property 3:
When the relationship is perfectly linear then Pearson's r will be ???1.
Note that properties 1, 2, and 3 suggest that Pearson's r will always be between—1 and +1, which is true.
Example 5 Revisited (Acrobat (PDF) 32kB Oct23 07)
Example 6 Revisited (Acrobat (PDF) 40kB Oct23 07)
In Example 5 there appears to be a perfect relationship (Y = X2), but the relationship is not linear and Pearson's r is not 1.
In Example 6, all the points are in Quadrants I and III; however, the relationship is not perfectly linear. Although the QCR is 1, Pearson's r is less than 1.
Property 4:
Pearson's r = ???1 if and only if the relationship between Y and X is perfect linear.
Pearson's correlation coefficient is a measure of the strength of the linear relationship between two statistical variables. Pearson's r does not depend on the units of measurement and will always between -1 and 1, inclusive. Note that an interpretation any correlation coefficient for quantitative data should be done in conjunction with an inspection of the scatterplot of the data.
When Pearson's r is positive (negative) this suggests a positive (negative) association.
When Pearson's r is close to 0 this suggests a weak linear relationship.
As Pearson's r moves away from 0 and gets closer to ???1, this suggests a stronger association.
A value of r close to either ???1, suggests a linear relationship.
A value of r equal to either extreme, ???1, will occur only if the points are all on a line.
Summary of Activity
This activity provides a developmental sequence for understanding Pearson's correlation coefficient. Pearson's correlation coefficient is a measure of the direction and strength of the linear relationship between two quantitative variables.