Using the Project EDDIE Prairie Eco Services in Environmental Systems
About this Course
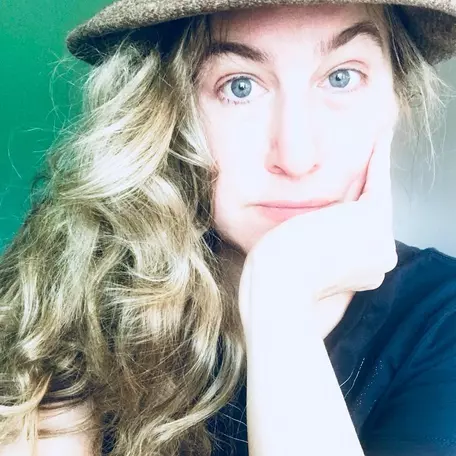
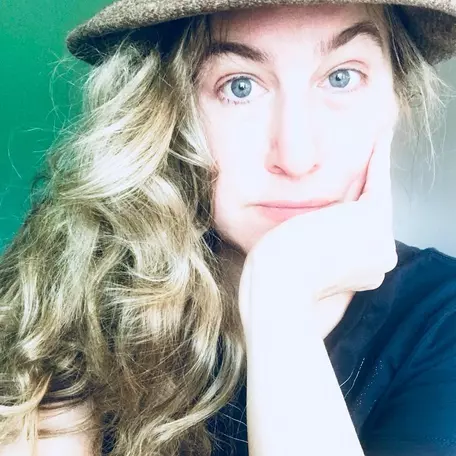
Environmental Systems
EDDIE Module Developed
This module allows students to explore quantitative reasoning/data analysis in a real-world context, by interpreting and modeling the data from the 2017 Hurricane Harvey flooding. Students also get to apply complex problem solving skills using design thinking, and environmental justice principles.
Jump to: Course Context | Teaching Details | Student Outcomes
Relationship of EDDIE Module(s) to my Course
This module was piloted in Spring 2020, as part of my Environmental System course's water unit. This unit covers water quality issues (pollution, salt intrusions, etc), flooding and droughts, inequitable access to clean water globally, and water treatment methods. Students' prior knowledge comes from content taught earlier in the course, including background on the hydrologic cycle, local watersheds and surface water features, biomes, and populations. Students were also given a brief "mini-lesson" on data analysis/statistics, and to think about how we can quantify the effectiveness of various flood mitigation techniques.
Teaching Details
What key suggestions would you give to a colleague before they used the activity in their teaching?
This is an activity that requires students to "think outside of the box", and as such, it is necessary to provide the appropriate context. This module uses one case study, which is for one location and is a single "snapshot in time". It's important to remind students that the impact of this model can be applied to a much broader scale, maximizing results. Also, I like to emphasize the environmental justice aspect, bringing in maps of redlined areas and how the most flood-prone areas, and the impacts of climate change (in general), are felt most by those in selected socioeconomic groups or those that are minorities/disenfranchised.
How did you address challenges in teaching with the module?
This is an activity best completed in pairs, allowing students to bounce ideas off each other and benefit from each other's strengths. Also, because some students might be approaching the lesson with limited modeling experience, I suggest that instructors run modelmywatershed on a Smartboard together as a class, demonstrating the various inputs/outputs, and walking them through a sample simulation. The introductory power point included in this module also provides the background information students need regarding the Hurricane Harvey case-study, and serving as a refresher for the characteristics that define a prairie ecosystem. Students not familiar with ArcGIS may also need 1-on-1 instruction with navigating overlays, delineating polygons, etc. This can also be done as a brief, whole-class tutorial.
Student Outcomes
This activity was highly effective in improving students' quantitative reasoning, Students were able to model a variety of preciptation/land use scenarios, and mathematically justify their conclusions. Students were able to justify their findings based off of their initial input values and "best fit" simulation run; more importantly, students demonstrated an increased ability to accurately interpret their data, and use it to make inferences towards new scenarios. This shows that the module systematically guides students through the problem solving process, using quantitative reasoning as key critical thinking skill.
This module also helps bolster student self-confidence in using large datasets. Based off of their pre-module and post-module evaluations (using a Likert scale self-assessment), most students reported feeling more comfortable manipulating large datasets (identifying outliers, plotting graphs, calculating trendlines, etc.), and more importantly, using large datasets to derive models of complex ecological problems. Anecdotally, students also showed decreased apprehension in later data-based or computational assignments, and appeared less "math-adverse" throughout the remainder of the course.